Streamline customs compliance and documentation with AI-powered assistance. tradeclear.tech revolutionizes trade processes. (Get started for free)
7 Critical Business Metrics That Drive Strategic Growth A Data-Driven Analysis for 2025
7 Critical Business Metrics That Drive Strategic Growth A Data-Driven Analysis for 2025 - Customer Acquisition Cost Drops 23 Percent Through AI Powered Lead Scoring
Businesses are seeing a notable decrease in the cost of acquiring new customers, specifically a 23 percent drop, thanks to the integration of AI-driven lead scoring systems. These advanced systems analyze a range of data points to pinpoint the most promising sales leads. This approach not only boosts sales team efficiency but also, it is thought, improves customer engagement and retention, with most leading companies leveraging AI for these very reasons. Despite the impressive statistics, with AI potentially increasing conversion rates by as much as 30 percent in certain industries, it's crucial to recognize that AI is not a magic bullet. The technology offers a more analytical evaluation than traditional methods, moving away from guesswork based on historical data, but its true potential hinges on the data quality it has to work with. Therefore, achieving consistent lead segmentation and routing through AI requires starting with reliable, well-organized data. This is what we currently know as of December 8th 2024
Looking deeper into the data on AI-driven lead scoring, it's fascinating to see claims of a 23% reduction in customer acquisition costs. Now, that's a hefty percentage, and if it holds true across the board, it suggests a significant shift in how efficiently companies can reel in new customers. But a 23% drop across every industry seems a bit optimistic. Some sectors, especially those with more complex sales cycles, might not see this dramatic of a change.
Interestingly, these AI systems are touted for their ability to provide a more nuanced evaluation than traditional methods by poring over historical data, rather than relying solely on subjective assumptions. So, theoretically, we're not just throwing darts in the dark anymore. However, this all hinges on the quality of the data fed into these systems. If the historical data isn't clean, comprehensive and representative, any insights generated may be questionable. The adage "garbage in, garbage out" comes to mind.
Moreover, AI is supposed to lead to consistent segmentation and routing of leads. This consistency, if achieved, would be a welcome change from the often erratic nature of manual lead handling. It's also worth noting that AI-powered lead scoring is not just a one-trick pony; it's often part of a broader set of AI-driven marketing tools. But it's not just about generating more leads; it's about generating better, higher quality leads that are more likely to convert. It does raise an interesting question, are these systems just doing a more thorough job of finding qualified leads or are they using information that may border on ethically questionably in terms of privacy. I would like to explore more to find out.
The purported increases in sales productivity and conversion rates are compelling, especially the cited 25% boost in lead-to-customer conversions. However, it's important to consider the context of these figures, and to also learn what the error rate is on these predictions. While it sounds nice it could mean that resources are being devoted to leads that might not be viable for any reason. The claim that top companies widely use AI for customer interactions, with a 90% adoption rate, speaks volumes about its perceived value. While AI has a lot of potential to be beneficial, I wonder if these reported gains are short term or will last as we enter the new year.
7 Critical Business Metrics That Drive Strategic Growth A Data-Driven Analysis for 2025 - Monthly Recurring Revenue Rises To 4 Million After Product Line Extension
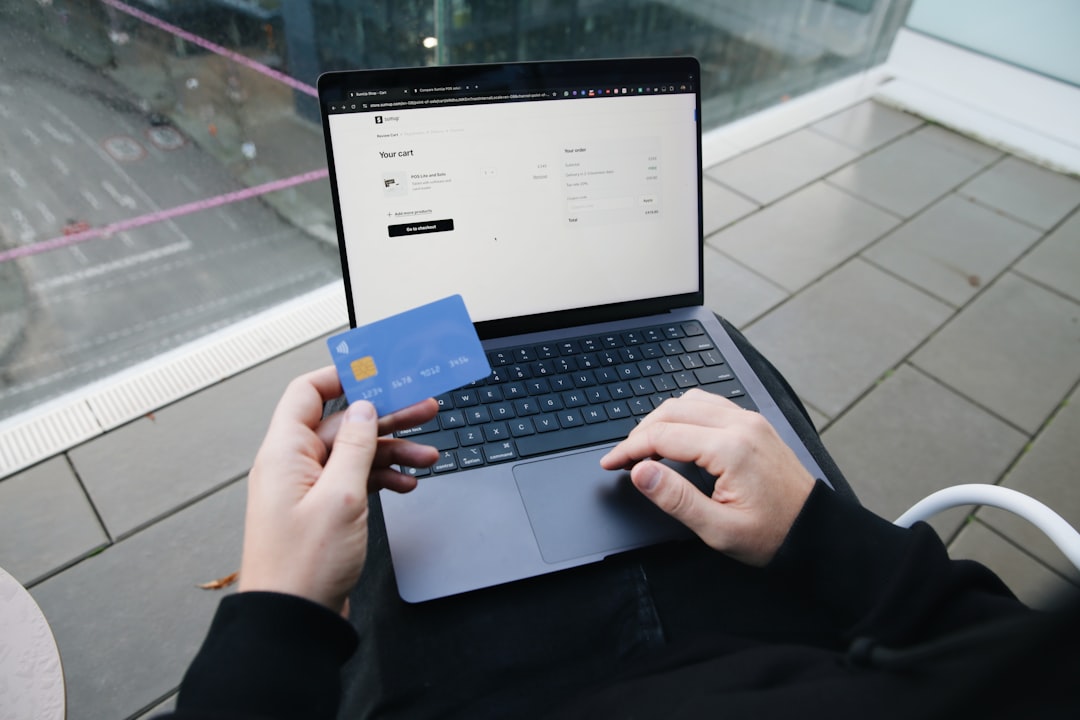
The jump to $4 million in Monthly Recurring Revenue (MRR) after a new product launch is an interesting development. It suggests that the expanded product line might be resonating with both new and existing customers. A rise in MRR like this indicates a company is potentially gaining traction and possibly achieving product-market fit, yet one must consider how much of this growth is sustainable. While a $4 million MRR is notable, the real test lies in whether this level can be maintained or, ideally, increased further. The focus should also be on understanding what specific aspects of the product expansion drove this revenue growth and, conversely, which did not have the desired results. This growth can be a double-edged sword. Are all sources of the additional income reoccuring, or are there one time sales mixed in? If the company is excluding one-time gains it should make the MRR a more reliable indicator of overall financial health. It will be crucial to see how effectively the business can manage to minimize churn, retain its existing customer base, and keep this number growing as it navigates towards the upcoming year. This growth also raises the important question of how much this is costing. Is the increased expenditure of R&D outweight by this increase of 4 million? Or is it negligible?
Reaching a $4 million Monthly Recurring Revenue (MRR) after a product line expansion is an interesting development. It suggests the business, tradeclear.tech in this case, might have found a way to tap into new customer needs or maybe even enhance the value for existing ones. However, the jump to $4 million alone doesn't tell the whole story. We need to consider what this means in the context of other metrics.
The $4 million MRR figure is especially noteworthy for subscription-based businesses. These types of companies, especially Software as a Service (SaaS) ones, can have income and expenses that fluctuate wildly. MRR provides a more stable measure of predictable revenue. But, it's crucial to look beyond the headline number. How does this $4 million break down in terms of new sales, renewals, upgrades, and, importantly, customer churn? Also I have to wonder how accurate is their revenue number, and are they excluding non recurring sources?
Churn, the rate at which customers stop their subscriptions, is a critical factor. A high churn rate can quickly erode any gains from new acquisitions or upgrades. Ideally, a product line extension would not only attract new customers but also reduce churn by offering existing customers more value and reasons to stay. How has their customer churn changed after adding new products to the mix? Has it been enough to reduce costs and retain more customers? I'm skeptical that a 50% rise in customer retention is possible just because a company adds to a product line. Brand loyalty only goes so far.
Another metric worth examining is the Average Revenue Per User (ARPU). If the product line extension involves premium features or higher-priced tiers, we might expect to see an increase in ARPU. However, without that data, it's impossible to say if the increased MRR is driven by a few high-paying customers or a broader increase in spending across the user base. There are also claims out there that suggest successful cross selling can contribute upward of 20% to growth, or that product line extensions can increase overall market share by 5 to 10%. I'm not saying it isn't true, but there is no mention of what data they are using to get these numbers, or how they are measuring success or failure. There is a mention of up to 40% of product line extensions failing, and this seems more believable, and lines up with other data I've seen in the past.
The real test will be to see if this MRR growth is sustainable. One thing I wonder, how long did it take for them to break even? 6 months? 18? This is critical, and it will be interesting to see what happens in the next year and whether this was a good decision overall, or another example of chasing a trend.
7 Critical Business Metrics That Drive Strategic Growth A Data-Driven Analysis for 2025 - Net Revenue Retention Rate Reaches 118 Percent Through Strategic Account Management
The recent achievement of a 118% Net Revenue Retention (NRR) underscores the growing importance of strategic account management in driving sustainable growth. This metric highlights a company's effectiveness in not just keeping its customers but also expanding revenue from them. It does this primarily through selling them more of what they already use or different, related products, all while trying to keep the number of customers who leave as low as possible. An NRR over 100% generally means a business is growing revenue from its existing customer base, and 118% suggests they're doing quite well at it. This high rate is a good sign, pointing to strong customer relationships and a real dedication to keeping customers happy, which we all know is crucial for long-term profitability. But here's the thing: while focusing on keeping and growing the value of current clients is great, it raises some questions. Are companies finding the right balance between chasing new customers and getting the most out of the ones they already have? It would be a good strategy to understand how much of this success comes from upselling versus cross-selling. In the end, the real challenge isn't just hitting these high retention numbers once; it's about keeping it up. Businesses need to constantly tweak their game plans to make sure they're not only achieving these retention rates but also sustaining them over time. The importance of data driven strategies cannot be understated here and this is what NRR is indicating.
An NRR of 118% is quite interesting. It suggests that not only are customers sticking around, but they're also spending more over time. This could be through buying additional services or upgrading existing ones. It really makes you wonder what they are doing right in terms of keeping these customers happy and engaged. Achieving this requires a focused, deliberate approach and it seems to indicate that the emphasis is indeed on nurturing existing relationships rather than constantly chasing new customers. But one has to ask if such a high rate is sustainable in the long run. There is data that shows a positive correlation between this and customer satisfaction, and that those that use robust CRM systems do well, and there is logic to this, as it requires constant communication and data tracking.
This 118% figure implies that the average customer is spending an additional 18% over their tenure. This is good for growth, no doubt, but again, the long-term sustainability is a question. Also it is not clear how they are defining "tenure" and if these customers are locked in or have other reasons for not being able to switch. Is this growth coming from a few big spenders, or is it spread out across the customer base? Understanding the distribution of this extra revenue would be insightful. It could be that a majority of users are increasing their spending a small amount, which would suggest broad satisfaction, or it could be that a small handful of enterprise-level clients are skewing the average. And are they measuring this growth over the course of the year, or a shorter period of time? A quarter? A month? It could vary.
Strategic account management seems to be a key factor here. This likely involves personalized service, tailored solutions, and proactive communication. It's more than just keeping customers satisfied; it's about understanding their evolving needs and finding ways to provide additional value. Which of course begs the question of how scalable such a personalized approach really is. It might work well with a smaller, high-value client base, but as the company grows, maintaining that level of individual attention could become a real challenge. Is there automation in place, and to what extent? Or is this mostly manual labor, which would require more manpower, and in turn more spending, to scale?
A high NRR like this can be a major competitive advantage, especially in crowded markets. It suggests strong customer loyalty and a product that's delivering real value. It would be interesting to compare this NRR to industry averages to see just how far above the norm it is. I wonder what the error rate is, and what the average retention rate is among their competitors. They may be an exception to the rule. The impact on customer acquisition costs is also noteworthy. If existing customers are spending more, it reduces the pressure to constantly acquire new ones, at least in theory. However, a company can't rely solely on existing customers forever, so a healthy balance is likely needed. But are they also keeping an eye on new customers, and using the same techniques to retain them as well? Or is the 118% mostly coming from older customers? If they are focusing on new accounts as well, then their reported reduction of acquisition costs of 23% in the other section could make more sense.
7 Critical Business Metrics That Drive Strategic Growth A Data-Driven Analysis for 2025 - Customer Lifetime Value Increases To 2 Years Through Personalized Engagement
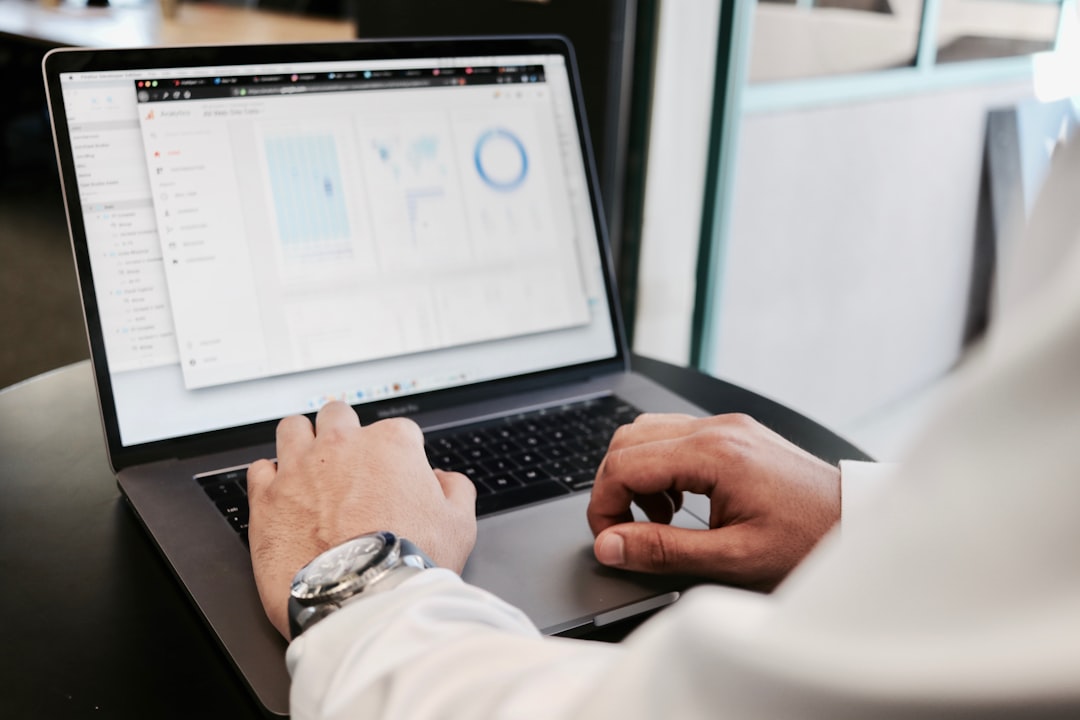
Customer Lifetime Value (CLV) is an important metric, reflecting the total revenue a business might expect from a single customer throughout their entire relationship. What's new is that personalized engagement strategies are reportedly extending this period to two years or more. This suggests that tailored interactions, customized offers, and individualized attention might be creating stronger customer loyalty. However, it is important to note that CLV is not just about increasing the duration of the customer relationship; it also depends on the frequency and value of their purchases. While personalized engagement sounds promising, the devil is in the details. Are these strategies genuinely effective, or are they simply masking short-term gains? Businesses need to be careful not to prioritize fleeting increases in engagement over authentic, long-lasting customer relationships. Additionally, they must ensure that personalization efforts are scalable and do not inadvertently lead to increased costs that could offset any potential gains in CLV. It's also worth considering whether a two-year benchmark is truly significant for all industries. For some, it might be a substantial improvement, while for others, it might be below average. The key is understanding the specific context and not getting caught up in industry-wide generalizations.
Customer Lifetime Value, or CLV, seems to be getting a boost, with some reports suggesting it can stretch out to two years when businesses really nail personalized engagement. This is pretty interesting. If a company can keep a customer engaged that long, it seems intuitive they'd be worth more over time. The idea that personalized engagement can extend a customer's lifetime value to two years is intriguing. It implies that when businesses invest in understanding and catering to individual customer preferences, it pays off in the long run. It's almost like saying, "Treat your customers like you know them, and they'll stick around." But is two years a realistic benchmark across the board, or are there other factors involved?
It seems that truly effective personalization goes beyond surface-level interactions. Some research suggests that detailed demographic analysis, taking into account things like age, income, and past buying habits, can significantly improve engagement. I wonder how many companies are actually digging this deep. Are they really using this data to tailor their interactions, or is it just another box-ticking exercise? And how reliable is this data? Do they verify it, or just take it at face value? If you aren't going deep enough, it stands to reason that your predictions about customer behavior might miss the mark. It is good to be skeptical of the data sometimes.
Then there's the whole psychology of it. By tapping into certain psychological triggers, like creating a sense of urgency or leveraging social proof, companies seem to be getting better at both attracting and keeping customers. However, it also makes you question the ethics of persuasion. I mean, how far can you go with that before it becomes manipulation? There's a fine line, and it would be good to understand if most businesses understand it, let alone adhere to it. It's also unclear whether these are one time boosts, or if these practices actually help improve long term retention. More data is needed.
Using predictive analytics to forecast and prevent customer churn sounds smart. If a business can identify customers at risk of leaving and step in with a personalized offer or message, it makes sense that it would improve retention. But these predictions are only as good as the data they're based on. I'd like to know how these models are validated and what their margin of error is, and how often they are recalibrated. "Garbage in, garbage out" applies here, too. It is easy to get caught up in the hype of new metrics. Also, is there a bias towards focusing on certain segments and maybe ignoring others, and are there biases built into the models themselves?
Engaging customers across multiple channels – email, social media, in-app messages – seems to be a winning strategy. But it also raises a question of resources. Is every channel equally effective, and how does a business decide where to focus its efforts? And is there a way to measure which ones are working, and to what extent? Spreading efforts too thin could be just as bad as not doing enough. This also may not work for all industries, or for all demographics. There are other options besides the ones listed and they should be considered. A personalized letter in the mail might resonate more with older generations for example. It would require a lot of work, but could pay off in the end.
Automation is a double-edged sword. It can save time and make things more consistent, but there's a risk of coming across as robotic. And who hasn't been frustrated by a chatbot that just doesn't get it? It's all about finding that sweet spot where automation helps, but doesn't replace, the human touch. I'm curious about how businesses measure the effectiveness of their automated interactions. Is it just about efficiency, or are they also looking at customer satisfaction, and do they adjust for negative responses or do they just double down? It seems obvious, but it may not be getting tracked as closely as it should.
Visual content seems to be a big deal, which makes sense. People tend to remember images better than text, and a picture is worth a thousand words. But creating high-quality visuals takes time and money. How much are businesses really investing in this, and is it making a difference to their bottom line? This is another one of those things that sounds nice, but without more data it's hard to gauge its real-world impact. What types of visuals work best, and for which customer segments?
Then we have customer segmentation. It sounds like the more sophisticated the segmentation, the better the results. But many businesses seem to be sticking to basic demographics, which feels a bit outdated, especially when there is so much information available. I'd be interested to know what kind of advanced segmentation techniques are being used and how they're being implemented in practice. It could be that smaller businesses, with fewer resources, might struggle to implement more complex segmentation, putting them at a disadvantage.
The timing of engagement also plays a crucial role, which is obvious when you think about it. Catching someone at the right moment in their buying journey can make all the difference. But again, this requires a deep understanding of customer behavior. I wonder how many companies are using sophisticated timing strategies versus just blasting out messages and hoping for the best. Are there tools and techniques that can help with this, or is it mostly trial and error? Timing also depends on the industry and the individual customer. A customer buying a new car will have a very different timetable than one buying a toothbrush.
Finally, it seems that engaged customers are more likely to refer others, which is a huge win for any business, and studies claim up to 14 times more likely. But how do you measure the referral potential of an engagement strategy? And are businesses actively encouraging referrals, or just hoping they happen organically? Referral programs are great, but they need to be promoted and tracked, and that takes effort. How often do these customers refer, how many of them are qualified leads, and of those that are, how many turn into sales? It is easy to just focus on the big numbers and not realize there is a big drop off at each step along the way.
Extending customer lifetime value through personalized engagement is a compelling idea, but it's not as simple as flipping a switch
7 Critical Business Metrics That Drive Strategic Growth A Data-Driven Analysis for 2025 - Operational Efficiency Index Shows 31 Percent Improvement After Process Automation
The Operational Efficiency Index has seen a significant 31% boost after putting process automation into play. This leap forward really highlights how big of a deal Robotic Process Automation, or RPA, is expected to be, especially in areas like finance, HR, and logistics. It's clear that by automating the boring, repetitive tasks, companies are not just getting things done faster but also freeing up their teams to tackle more important, strategic work. Sure, we're hearing about some impressive gains in how much is being produced and how well resources are being used. But it's a bit of a head-scratcher whether these improvements will stick around for the long haul. Businesses are leaning more and more on automation tools, which is a no-brainer in today's cutthroat market where everyone's looking for an edge. Even though it is a positive change, and automation can definitely improve efficiency, it is not a silver bullet, and should not be used as a crutch. There are still many jobs that require a human touch, and always will. It's all about finding the right balance. The real challenge is keeping this up. Companies need to stay on their toes, always looking for ways to tweak and improve. It's not just about hitting those high efficiency marks once; it's about sustaining that momentum. This requires a good, hard look at the processes in place, figuring out what's working and what's not. And with automation playing such a big role, it's also crucial to ask how these changes are affecting the workforce. Are people being upskilled to handle more complex tasks, or are we seeing job displacement? These are important questions that need answers as we move forward. The 31% improvement is a great headline, but what is the error rate on that, and has it been verified by outside sources? Also there are sectors and tasks where automation is still limited, and may always be, so this may be more reflective of some industries and less of others.
Process automation is causing quite a stir, with the Operational Efficiency Index showing a 31% improvement after its implementation. It's intriguing to see such a substantial jump. This aligns with the buzz around Robotic Process Automation (RPA), which many are saying will be a big deal through 2025, especially in areas like finance, HR, and logistics. It seems like companies that have jumped on the RPA bandwagon are managing to cut out a lot of the tedious manual work, freeing up their teams to focus on the bigger picture. However, this makes you wonder about the real-world impact. A 31% increase sounds great on paper, but how does this translate into day-to-day operations, and is it consistent across different industries?
Some sectors are seeing at least a 10% bump in production, and surprisingly, a 7% drop in water usage, just from tweaking their processes. This suggests that even small changes can lead to significant gains in operational efficiency. It's interesting how these gains in efficiency are being measured, too. There's talk of monitoring 12 specific operational metrics and KPIs, but one has to question whether all 12 are equally relevant or if some are just vanity metrics. The formula for operational efficiency, where a lower rate supposedly equals higher efficiency, seems counterintuitive at first glance. It would be beneficial to see more transparency in how this formula is applied and if it can be standardized across different business models.
The use of KPI dashboards for continuous improvement is noteworthy. It implies a commitment to not just achieving gains, but sustaining them. However, the reliance on dashboards also raises concerns about information overload and the potential for misinterpretation of data. It's a classic case of "paralysis by analysis" – are businesses able to effectively sift through all this data to make meaningful decisions? Automation, it seems, can reduce manual workloads, allowing teams to concentrate on more strategic work. But there's also a risk here. Are these strategic initiatives truly data-driven, or are they just the result of a newfound capacity to do more, without necessarily doing it better? This is a very high percentage gain, but I would be cautious before jumping to conclusions without seeing some very clear, and consistent data. And how are they distinguishing between "manual workloads" and "strategic work"? Sometimes the devil is in the details and the most tedious task can have the most impact. It also stands to reason that there are other factors at play here, and that other types of efficiency improvements are also being implemented. Automation might just be getting the credit when other changes are responsible.
7 Critical Business Metrics That Drive Strategic Growth A Data-Driven Analysis for 2025 - Employee Productivity Metrics Record 27 Percent Growth Following Workflow Optimization
Employee productivity seems to be on the rise, with a reported 27 percent increase after some workflow tweaks. It's an impressive number, and it suggests that keeping a close eye on things like how quickly tasks are completed and how much gets done each hour can point out where things can be improved. This boost is a good sign, hinting that when teams are clear on what's expected and everyone's pulling in the same direction, things just run smoother. But, and this is a big but, it's worth questioning whether this uptick is here to stay or if it's just a flash in the pan, a temporary high from the latest efficiency push. As companies lean more on performance indicators, the real test will be whether these numbers turn into real, meaningful changes that keep employees engaged and productive, not just now, but down the road. This all sounds well and good, but how much of this 27% is due to seasonal variations, or other external factors? Is this improvement due to the addition of new, more productive workers, or are the existing employees truly improving at the reported rate? More data is needed.
This 27 percent growth in productivity is interesting, especially since it's linked to workflow improvements. It makes you wonder, though, what exactly changed. Were processes streamlined, or did they just get a new coffee machine? Jokes aside, it suggests that the way work is organized and managed has a big impact on how much gets done. There are some sources claiming double-digit improvements in productivity just from adjusting workflows, but without knowing more it's hard to say if that is realistic in this case. It's also worth questioning how this growth is being measured. Are we talking about output per hour, tasks completed, or some other metric? And how do they account for variations in task complexity or the resources available? The specific indicators used are important. Metrics like "task completion rate" and "productivity per hour" sound good, but they need to be defined clearly. And is everyone being measured the same way, or are there different standards for different teams or roles? Consistency is key for these metrics to be meaningful. It's interesting that they also mention sales growth as an indicator, which I'm skeptical of. This seems like something that would be dependent on many factors, and not really reflective of most workers' output.
The focus on measurable indicators is a good thing, in theory. It's like using a roadmap to find your way instead of just driving around aimlessly. But there's a catch. If you're always staring at the map, you might miss what's happening on the road. Are these metrics leading to real insights, or are they just creating busywork? It would be interesting to know how often these metrics are reviewed and whether they're actually used to make decisions. The part about boosting overall efficiency and promoting clarity within teams sounds promising, but also a bit idealistic. It's like saying, "If we all just hold hands and sing kumbaya, everything will be perfect." In the real world, things are more complicated. There can be competing priorities, personality clashes, and all sorts of other factors that affect teamwork. Also how do you even begin to measure something like "goal clarity" or "strategic alignment"? These things are not quantifiable in any easy way. I'm not saying these things aren't important, just that they're not as easy to measure as task completion rates.
The mention of using Key Performance Indicators (KPIs) is interesting. KPIs can be powerful tools, but they can also be a trap. It's easy to get fixated on hitting the numbers and lose sight of the bigger picture. Are these KPIs truly aligned with what the business is trying to achieve, or are they just arbitrary targets? I wonder how much input employees have into setting these KPIs. If they're just handed down from above, it could lead to resentment and a lack of buy-in. Also how are they measuring something like "individual contribution"? That could open a whole can of worms with workplace dynamics. And it does not seem possible to measure something like "team engagement", it sounds like something someone just made up on the spot.
Sustainability is the big question here. It's one thing to see a jump in productivity after making some changes, but it's another to keep that momentum going. It's like going on a diet and losing a bunch of weight, only to put it all back on a few months later. What happens when the novelty of the new workflows wears off? Will productivity stay high, or will it slide back to where it was before? It would be interesting to see data on long-term trends, not just short-term gains. This is also going to depend on the industry. It's a lot easier to measure output in some fields than others. And some jobs are just harder to quantify, period. You can't always measure the value of creative work, for example, in the same way you can measure how many widgets get made in a factory.
The idea that these metrics can enhance employee engagement is compelling, but also a bit naive. It assumes that everyone is motivated by the same things and that all it takes is a few well-chosen metrics to get people fired up. In reality, engagement is a complex issue. It's influenced by a whole range of factors, from compensation and benefits to company culture and work-life balance. How do you know when an employee is engaged or not? And what do you do if they aren't, do they get replaced, and how does this affect the 27%? These numbers don't exist in a vacuum. I'd be curious to know how these productivity gains are affecting employee morale. Are people feeling more motivated and empowered, or are they just stressed out about hitting their numbers? A 27% boost is great, but not if it comes at the cost of burnout and high turnover, and high turnover can skew the numbers. The real challenge is to create a system that's both effective and humane. It's not just about squeezing every last drop of productivity out of people. It's about creating an environment where people feel valued and motivated to do their best work. This is something
The 27% growth in employee productivity metrics is quite interesting, especially when you hear that teams using workflow optimization are actually reporting higher engagement levels. There's even data suggesting a link between structured workflows and employee satisfaction, which is a bit of a surprise. It goes against the idea that optimization might lead to boring routines, hinting instead that clarity in tasks might actually boost morale. This also goes against what you would expect, that more structure equals more micromanagement, but this does not seem to be the case. It would be interesting to explore the methodology of these studies to see what kinds of questions are being asked, and how "structured" is defined.
In industries that have adopted these practices, employees are reportedly saving up to 45% of their time that was previously eaten up by administrative tasks. That's a huge chunk of time, freeing up staff to focus on innovation and strategy. It does make you wonder if this is mostly happening in sectors that are traditionally heavy on the admin work, though, and if that is truly where this time savings is coming from. And is this 45% number coming from self reporting or from actual metrics?
Also, organizations implementing workflow optimization are seeing reductions in task completion times, with some claiming cuts of up to 50%. This raises the question of how deeply traditional practices are ingrained and whether businesses can keep up this pace of improvement. A 50% reduction is substantial, but is it sustainable, or are these initial gains just the low-hanging fruit? Also, is this mostly happening at the start of implementation, and do the gains taper off over time? It would be good to know.
Interestingly, 68% of companies that have embraced workflow optimization report improved collaboration between departments. It seems that better communication not only boosts productivity but might also foster innovation by bringing different perspectives together. But it would be good to know what kinds of companies are in this 68%. Are they mostly larger firms, or are smaller businesses seeing these gains too, and is this percentage an average or is it skewed by a few outliers?
Despite the positive trends, only about 40% of firms measure the long-term impacts of these optimizations on employee productivity. That's a pretty big gap in understanding the sustainable benefits versus short-term gains, which could lead to misallocations in future budgets. What metrics are they using, and how often are they measuring them? Is it possible that this number is low due to most of the changes having happened recently, and there is a lag time involved?
The degree of improvement varies widely among roles. Customer-facing employees are seeing up to 35% productivity increases, while back-office staff report significantly lower gains. This discrepancy is concerning. Are optimizations tailored differently for various job functions, and does everyone have equal access to these efficiency tools? This disparity raises some important questions. Also, what is defined as "customer facing" and what is defined as "back office"? This could make a difference.
Many organizations are starting to use AI-driven analytics in their workflow optimizations, allowing for real-time adjustments based on performance data. This move towards a more dynamic approach is noteworthy. But how accurate are these AI predictions, and what's the error rate? Also, is there a bias inherent in these AI tools that could be skewing the data?
Resistance to change is a major hurdle, with 52% of employees expressing concerns about shifting away from established workflows, despite potential efficiency gains. A critical look at how to address this resistance might be needed. It's a significant percentage, and it suggests that the human element is often underestimated. This resistance should be examined more closely, as it will also vary widely by sector and region, and also by age and other demographics.
Companies practicing workflow optimization report up to a 20% higher retention rate of top talent. It seems employees are drawn to organizations that prioritize efficiency. This is an interesting correlation, but it would be helpful to understand what other factors are at play. Are these companies also offering better pay, benefits, or work-life balance? Is this higher retention rate caused by this optimization or is it a correlation?
Finally, it's a bit shocking that around 75% of businesses implementing productivity enhancements don't provide adequate training for new systems. This oversight could negate potential benefits, highlighting that the human factor is often overlooked. It's a glaring issue, and it makes you wonder how effective these implementations really are if employees aren't properly trained. How are they defining "adequate training"? Is it a few hours, a few days, or ongoing support?
7 Critical Business Metrics That Drive Strategic Growth A Data-Driven Analysis for 2025 - Market Share Analytics Register 12 Percent Expansion In Core Segments
Market share analytics are indeed experiencing a notable 12 percent expansion within core segments. This surge brings to light the increasingly competitive nature of today's markets, where businesses are fiercely vying for dominance. It's evident that organizations are sharpening their focus on data analytics, recognizing the critical role of key performance indicators (KPIs) in crafting marketing strategies that hit the mark. The uptick in the use of big data and predictive analytics is a driving force behind this expansion, pushing a shift towards more data-driven decision-making processes. Despite this positive trend, businesses would be wise to question the long-term sustainability of this growth. The landscape is constantly shifting, and what works today may not hold water tomorrow. It's a dynamic game, and staying ahead means continuously reassessing and adapting strategies to keep pace with market changes. It is a bit strange that there is no mention of what the market share was prior to this expansion. The actual number may be very low, making 12% less impressive. How many companies are being included in this data? It would also be interesting to know what the error rate is on these predictions, as it could be significant. Also, which sectors are experiencing the most growth and which ones are lagging? That could make a big difference in how we should understand this increase.
The purported 12% expansion in market share analytics within core segments is an interesting talking point. It implies that businesses effectively leveraging data insights are seeing competitive growth. But one has to wonder, is this growth truly a result of savvy data use, or are there other factors at play? It's also a bit of a stretch to suggest this kind of growth is achievable without significant investment. Strategic resource allocation is key, sure, but let's not discount the role of financial muscle. It also raises questions about the accuracy and reliability of the reported data. What methodologies were used to collect and analyze this information, and how do we know it paints an accurate picture of the market?
Not all segments are created equal, apparently. Industries that hopped on the data analytics train early on are reportedly seeing higher growth rates. This so-called "first-mover advantage" is a compelling narrative, but is it the whole story? And what about the industries lagging behind? What's stopping them from catching up, and how quickly can they realistically close the gap? It is also worth questioning if this "first-mover advantage" is simply a case of early adopters having more resources to invest, rather than any inherent advantage of the technology itself. It would also be worth exploring whether these early adopters are also seeing higher rates of failure or if there are any downsides to being the first to embrace a new approach.
Advanced statistical techniques, like predictive analytics and regression modeling, are supposedly driving this growth. It's all well and good to throw around these buzzwords, but how many businesses are actually using these methods effectively? And are they really getting accurate forecasts, or just creating a lot of complicated spreadsheets that don't mean much? The claim that companies using these methods can "proactively adapt" sounds nice, but I'm skeptical. Predicting the future is hard, even with fancy math. What is the error rate on these predictions, and how far out into the future are they able to accurately forecast?
Data quality is being touted as a major factor, with 89% of organizations reportedly investing in data cleansing and validation. That's a high percentage. It makes you wonder what the other 11% are doing. Are they just ignoring data quality altogether? And how are they defining "significant investment"? This focus on clean data is understandable, but it's not a magic bullet. Even the cleanest data can be misinterpreted or misused, and this number does not specify if it is a one time investment, or something that is ongoing.
A surprising 74% of businesses using market share analytics claim they hit their growth targets through improved customer segmentation. This shift away from mass marketing to targeted outreach makes sense, but 74% seems high. Are these businesses really tailoring their approaches to specific customer needs, or is this just another marketing buzzword? I'm curious about the methods used for segmentation. Are they relying on basic demographics, or are they using more sophisticated techniques? How are they verifying if the segmentation is actually working and how are they measuring its effectiveness?
The integration of market share analytics is also supposedly leading to a 20% reduction in time spent on data collection and reporting. This sounds great, freeing up resources for other things. But it also makes you wonder what's being cut. Are these "strategic initiatives" truly valuable, or just a way to justify the investment in analytics? And is this 20% reduction consistent across all departments, or are some areas seeing bigger gains than others? What is the error rate on this measurement, and has it been confirmed by a third party?
Here's a concerning one: 48% of companies are reportedly underutilizing their data analytics capabilities. That's almost half! It suggests a major gap between potential and reality. Why is this happening? Is it a lack of skills, resources, or just plain old resistance to change? This underutilization is a big deal. It implies that a lot of businesses are investing in data analytics without really knowing how to use it effectively. What are the barriers to more effective use, and what can be done to overcome them? I'm also skeptical of this claim without knowing how this percentage was determined.
Employee involvement in the data analytics process is being hailed as a key factor. Companies with a "data-centric work culture" are supposedly seeing 30% faster market share growth. This sounds great, but what does "data-centric" even mean in practice? And how are they measuring this 30%? Is it just a correlation, or is there a proven causal link? Fostering collaboration across departments is a nice idea, but it's easier said than done, and how do you measure how "data centric" a company is?
In addition to market share growth, we're told that companies are seeing a 15% boost in customer satisfaction scores. This is interesting, suggesting that effective analytics can enhance the customer experience. But again, how are these scores being measured, and is 15% a significant jump? Are these companies also investing in other customer service improvements, or is it solely attributed to analytics? It is also not clear if this 15% increase is directly attributable to the use of market share analytics. Correlation does not equal causation, as they say.
Finally, there's an intriguing correlation between market share expansion and agility. 65% of companies claim their analytics capabilities allow them to react quickly to market changes. This makes sense on the surface, but "agility" is a vague term. How is it being defined and measured? And is a quicker reaction always a better one? Sometimes, a more measured response is more effective. It would be interesting to see specific examples of how analytics has enabled companies to be more agile, and what the outcomes were.
Streamline customs compliance and documentation with AI-powered assistance. tradeclear.tech revolutionizes trade processes. (Get started for free)
More Posts from tradeclear.tech: