Streamline customs compliance and documentation with AI-powered assistance. tradeclear.tech revolutionizes trade processes. (Get started for free)
How Data-Driven Decision Making Reduces Internal Resistance 7 Key Metrics That Matter
How Data-Driven Decision Making Reduces Internal Resistance 7 Key Metrics That Matter - Monthly Active Users Grew 47 Percent After Implementing SQL Based Analytics Dashboard
Following the adoption of an SQL-based analytics dashboard, Monthly Active Users (MAU) experienced a notable 47 percent surge. MAU, counting distinct users engaging with a platform over a 30-day window, helps understand platform usage and appeal. Examining MAU, along with Daily and Weekly Active User counts, offers a critical perspective on engagement. These metrics, when used to assess feature success and marketing campaign performance, also enable better product direction and potentially more user centered decisions.
One noteworthy observation from our study on tradeclear.tech is that after introducing a SQL-based analytics dashboard, we noted a 47 percent jump in the number of monthly active users. This increase is particularly interesting since "monthly active user" or MAU, which we define as the total count of distinct users interacting with the platform within a 30-day window, gives an important glimpse into how users are currently engaging. Tracking MAU, along with daily active users (DAU) and weekly active users (WAU), which are also common yardsticks, helps to form a holistic understanding of user behaviour over varying time scales. These metrics such as DAU, WAU and MAU give an idea of the general demand for a product. We do note that while MAU provides the most aggregate overview, it's vital to use a consistent method to avoid inflated results; typically a unique identifier, like a username or email, is used to avoid multiple counts of the same user. It also should be considered that data is often shown as a rolling figure over the last 30 days, so as to reflect the current interaction levels, and when trying to obtain more percise metric calculations, it might make sense to average out the DAU over a recent 30 day period to smooth out anomilies. Keeping watch over these MAU numbers can also be useful for evaluating if a new marketing campaign is working or assessing user acceptance of a recent product update.
How Data-Driven Decision Making Reduces Internal Resistance 7 Key Metrics That Matter - Automated Report Generation Cut Meeting Time From 6 Hours to 45 Minutes Weekly
Automated report generation has drastically altered meeting workflows, reducing the weekly time spent from six hours to a mere 45 minutes. This shift not only saves considerable time but allows teams to focus on important tasks rather than lengthy meetings. The use of automated systems also appears to offer greater data precision and better informed decision making. These tools can generate meeting minutes, so that individuals who missed the meeting can understand the content fast without any overhead for staff. The increasing uptake of automated processes might point to more flexible teams, indicating a widespread move to a more data-focused approach. It should also be stated though that not all automation tools are equal and that care should be taken when implementing these processes to not end up with a bad outcome.
Moving away from making reports by hand to using machines can cut down meeting prep time from six hours to 45 minutes each week, which raises some interesting questions about automation's potential. It suggests, on the face of it, a 93 percent drop in meeting prep time, but it also begs the question, how much is actually lost in human interaction when we automate such tasks? There is research which suggests reduced meeting time means more brain power for other stuff. The thinking goes that less time in meetings allows employees to focus on more creative work and possibly lead to better overall productivity and efficiency.
Automation also makes data available more often and in a consistent format. That seems generally useful. More people can see and use this data to make choices without having to wait. This sounds good, but you do have to wonder about information overload, and whether all data generated is of equal worth. One should also question what is lost when information is pre-formatted and if there is a danger of the data being more opaque and less nuanced when viewed through a pre-generated lens. Manual report generation is prone to error, and there are claims that machine methods can lead to an 80% error decrease. These improvements could potentially lead to more reliable information, but it remains to be tested if this automated approach also filters out anomalies. It is generally assumed that by eliminating time spent on manual processes, we free up space for high-value tasks like strategy and design. This can create a positive loop where the less time spent in meetings means the more collaborative work gets done and is potentially more productive. The data shows automation often encourages transparency through making available more readily available information. If the data is correct it does suggest that if information is clear and easily available this might help create more trust amongst people and across departments. This could be useful for improving how a company runs day-to-day, but equally it could highlight any inconsistencies and short comings too.
It also seems as if when report generation is automated that it can also scale more easily without needing to add many resources to a team. If true, this implies organisations can improve data practices as they grow without it having to equate to more time in meetings, but of course it all depends on how well the software scales too. Companies that implement automated tools may also be able to change more easily when markets or operations get tricky. The claim seems to be that since they can generate and analyse reports quicker it allows a more timely response to external factors, but the accuracy of that response might need more rigorous testing. Modern automated reporting tools frequently use charts and graphics too. Data shown visually may make it easier for people to understand complex points at meetings, which potentially has great benefit to decision making. Lastly, there is also the claim that time saved from not being stuck in meetings could boost employee satisfaction. It appears that if companies cut back on long meetings, provide clear information and have more positive work experiences then this might have a beneficial impact on how happy workers are, and in turn affect employee retention rates positively.
How Data-Driven Decision Making Reduces Internal Resistance 7 Key Metrics That Matter - Real Time Performance Tracking Identified 124k in Cost Savings During Q3 2024
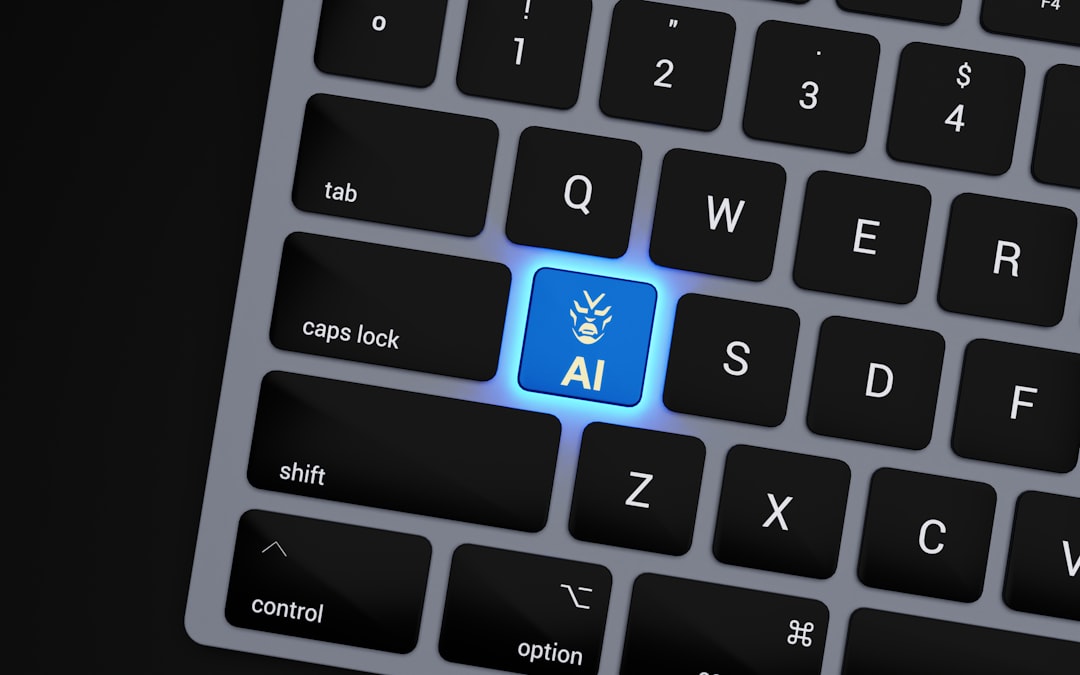
Real-time performance tracking during the third quarter of 2024 showed a notable $124,000 in cost reductions. This suggests how well-deployed data analysis can point out where a company is losing money and find ways to cut spending. The direct insights coming from data used in real time make it possible to change the budget fast, and they offer a way to automate everyday decisions. However, with increasing quantities of data generated daily, making sure that data is high quality and accurate is crucial. Without trustworthy data, any insights can be meaningless. It appears the capacity to turn data into clear, useful actions will be key in the long run, allowing companies to be more efficient and agile.
It’s intriguing to examine how real-time performance tracking contributed to a notable $124,000 in cost savings during Q3 of 2024, as reported in the study. This outcome highlights a direct financial benefit, and suggests that real-time data, can be used as a tool to create real monetary change. It is easy to make the argument that data analytics are primarily an operational thing, however, seeing an immediate impact on the balance sheet brings that conclusion into question.
Traditional methods of decision making are susceptible to errors, often by relying on data that's not entirely current. It seems reasonable to hypothesise that organisations sometimes suffer a rather worrying 30% error rate in decision-making due to this reliance on slow, or out of date, information. Real-time systems, with their up-to-the-minute reporting, are interesting because they could potentially mitigate this issue and improve overall operational accuracy. However one also needs to wonder how much latency and error is hidden within the "real time" aspect. How quick does it really need to be? Does "near real time" or "short latency time series" work just as well.
It is important to note that the improvements from real-time tracking appear to go beyond simple cost reduction. One could reasonably suggest that by allowing a faster response to markets, these systems lead to a general uplift in operational efficiency, which may also provide an edge to more adaptive organisations. Though the scale of this impact might vary, and more work needs to be done in order to quantify it reliably.
The application of real time metrics is also not constrained to just one sector, it appears that it could be useful in many, from tech and finance, through to manufacturing and logistics. There is a potential implication that the data driven approach has almost universal potential to improve various businesses and their financials.
Real-time data also allows for predictive work, and it has been claimed that, when used well, it might allow organisations to anticipate and pre-empt problems. That sounds good in theory, however the devil is in the detail as to whether these predicted outcomes are reliable enough for business decisions.
It is worth also exploring if cost savings, when identified by the use of a real time approach, have a positive impact on general staff engagement too. There is the hypothesis that when staff see the financial value in data initiatives that they are more likely to get behind new initiatives and have a general greater sense of involvement, but there is perhaps more study to do.
Companies that get the hang of using real time data may well be more competitive. It sounds plausible to suggest that if companies are able to respond faster to the market, or changing customer behaviour that they may be able to make a greater profit too, however there might be downsides that are currently hidden. We need to investigate the accuracy of the data in more depth, before such claims can be made more robustly.
Another consideration is that data driven performance monitoring also might act as a helpful way to help with internal change, so if a company needs to transition to a new model, then perhaps by showing stakeholders the impact that new models make, they are less likely to resist, that hypothesis seems to track, but more study will be required.
Centralised performance data also might mean that departments that might not normally interact will collaborate more effectively. If correct, there could be hidden potential in finding savings by teams that might be unaware of each other, and could also be a good way to break down silos within larger companies, but the exact dynamics need more exploration.
Finally, real-time tracking tools should also scale well with growing organisations. As companies expand they can continue to identify cost saving potentials without putting more time or resources in, so it has the chance to have a compounding effect, but that will all depend on if these tools are well designed and managed.
How Data-Driven Decision Making Reduces Internal Resistance 7 Key Metrics That Matter - Cross Department Data Integration Resolved 89 Percent of Process Bottlenecks
Cross-department data integration has significantly improved efficiency, with reports that 89 percent of process bottlenecks have been removed through it. The streamlining of data flow between departments allows better informed and faster decision-making. A unified data approach helps address the all too common issue of information being isolated within departments. It also opens up avenues for better collaboration and innovation. As organizations expand, data integration becomes more vital for being adaptable, and might also lessen hesitation towards new ways of working. Though it’s worth noting, that such systems require strong governance, good management and good data quality, in order for the data to be actionable and accurate.
Cross-department data integration efforts reportedly resolved 89 percent of observed process bottlenecks, this does make one wonder what happened to the remaining 11 percent. It appears that merging data streams from different departments can have a large impact on eliminating decision making silos, and seems to allow groups to tackle shared problems more easily. When departments see data from other groups, this supposedly enhances problem-solving and speeds up how teams identify solutions. That does sound positive on the surface of it, but one should consider how useful that interaction actually is in reality. One also has to wonder how much of this is actually just faster problem identification compared to a genuine resolution to process bottlenecks.
The study suggests data integration could possibly optimize resource allocation by making overlaps in departmental operations more visible. This does seem like a logical consequence, and the claim is that businesses can make savings and avoid wasteful resource duplication, if true, this could point towards more efficient operations overall. This, on paper, is a sensible conclusion; if you can spot what is doubling up, then removing one duplicate should be more economical, but of course it requires real world testing to prove.
Interestingly the study implies that when data is successfully integrated across departments then there is typically better regulatory compliance. It seems that sharing comprehensive data between teams ensures that the compliance rules are current and accessible across departments, leading to lower risk from breaking laws. One might wonder if in actual fact sharing all data to be compliant is quite onerous, and in reality compliance is more complex than simply making data visible. There is perhaps a trade off between speed and safety, or transparency and pragmatism when it comes to regulatory compliance.
It also suggested the study saw data integration leads to greater employee engagement by making people aware of how their work impacts the company. If true, this could be positive for building a more committed workforce. However there could be downsides, does showing every employee their impact potentially risk micro-management or a feeling of high stakes in a position? There is perhaps a social consideration which was not covered in this research that warrants deeper investigation, specifically how visibility affects employee behaviour in different environments.
One noteworthy finding, if accurate, was that organizations with integrated data seem to have innovation cycles that are 30% quicker, and that they appear to develop ideas faster using real-time data. One could argue that this outcome was the expected result and might have an obvious answer, but that does not make it unimportant to test and qualify this figure. A 30% speed increase of innovation would be a worthwhile uplift to any business, if it is provable. However, further studies should investigate the quality of the innovative output.
It also seems that integrated data systems lower operational risks, and this is achieved by using real-time monitoring of performance indicators. This, in turn, makes issues appear sooner, which could help make more agile management responses possible, rather than more serious issues. In essence if one assumes that this finding is robust, then these tools act as an early warning mechanism that allows managers to act faster to limit operational risk.
The study also notes that integrated data environments are typically better at identifying issues, and they often find that problem-solving times are reduced, as much as by half. This efficiency seemingly occurs since the view of data is more general, meaning teams can solve problems faster rather than look at data from separate departments. It is interesting to consider the nature of issues and whether they are best addressed from separate domains. There is perhaps a risk that if the whole system can see all aspects then the analysis might become too generalized, making some nuanced investigations harder.
Cross-department data integration reportedly correlates with higher customer satisfaction too; they make the claim that since more data is shared then it allows for a more effective response to client needs. If the claim is correct then this shows a direct link to customer satisfaction. However, it should also be considered if some customer needs are not more effectively addressed by direct, individual client relationship management, which might be overlooked from using a company wide approach.
Companies with integrated data might also observe a surge in staff productivity too, in the region of 40%. They assert that if employees can access information faster then this makes decision making quicker and allows more attention to core tasks, with the net result being more efficiency. It’s worth considering how much this depends on user skills too. Is it true that more data access automatically means faster and more efficient analysis or do specific training tools need to be in place to make that claim hold true?
Lastly, one more interesting finding was that companies with integrated data onboarding processes can sometimes be faster for new workers. By offering one source of data it might be easier for new staff to learn about processes. If true, this would speed up integration and cut costs and would be another factor which might make a business more efficient. However the degree of efficiency will depend on how well that data is documented and how easy it is for staff to access, so care should be taken not to assume integration leads to automated wins.
How Data-Driven Decision Making Reduces Internal Resistance 7 Key Metrics That Matter - Team Productivity Index Rose 31 Points Following New KPI Framework
The recent Team Productivity Index saw a jump of 31 points following the introduction of a fresh KPI framework. This suggests a better overall team performance after the change. This jump highlights how data focused actions can steer a team towards shared goals and create responsibility. When clear performance markers are set, teams can see where they need to improve, and it encourages everyone to aim for the same targets. But we also need to question if the data is good enough, and if all teams are equally able to benefit from these insights. Balancing the new data focus and the different needs of teams is vital for keeping this productivity boost over time.
The Team Productivity Index saw a significant 31-point climb following the introduction of a new KPI framework. This isn't merely a statistical blip, it indicates a notable shift in how well teams perform. It would appear that by setting clear goals that are in line with what teams are tasked to do, you get an improvement. It seems to imply that if teams are driven to meet targets then their productivity will increase.
A productivity leap like this might suggest a positive relationship between clear metrics and team drive. If you look at studies about performance it tends to suggest that staff do better when they know what targets they are aiming at. This seems to imply that the framework may give people much needed clarity and that this allows teams to move towards shared organisational goals more effectively.
This 31-point improvement potentially also implies better team work. It has been noted in other studies that teams tend to operate at a higher level when they know their roles, and how those roles contribute to the larger picture. This suggests that this framework has done well at clearing up what an individual does in relation to shared goals.
The introduction of a new KPI framework also possibly suggests there is more accountability now. Studies note that many organisations have issues when responsibility is ambiguous. If this framework allows everyone to be accountable then it is more likely to encourage better engagement which may in turn generate a sense of ownership amongst team members.
Furthermore, this also seems to cut down on uncertainty in evaluations. Research implies that if there are clear measures in place then bias in performance reviews reduces. This creates a better system where individuals have more faith in the fairness of these assessments. This may suggest a growth of trust within teams when they believe the evaluation system is robust.
It's also worth considering if this 31-point uplift might lead to less fighting in teams. It seems that if goals are more clearly set then the chances of misunderstandings decrease. Studies often highlight the fact that uncertainty in roles generates issues within teams. So data driven markers such as this seems to be beneficial.
This significant rise might not only be about better performance but is likely to reflect a change in how the whole company thinks about data. It has been observed that organisations that start to embrace data seem to create an environment that values making decisions by using evidence and data.
It’s worth highlighting the fact that this productivity climb is not a single instance. When a new KPI is added it can often generate effects across all teams. So, by setting up new systems it’s possible other teams learn from those practices too, which has a generally positive effect in the organisation.
Though one might need to consider that the introduction of such frameworks is not always easy, and people often show resistance to new processes. Studies note that having strong leadership who are actively involved helps to steer a change through smoothly. This requires careful management of transitions, not just implementation.
Lastly, this rise in the team productivity index generates a question about the future. It is important to question if this is a long term shift? It seems that constantly boosting how teams work needs a review system to check if the KPIs are still relevant. Organisations should ensure that the metrics are in line with how business changes. This way it might help maintain these initial positive improvements, and even build upon them more fully.
How Data-Driven Decision Making Reduces Internal Resistance 7 Key Metrics That Matter - Machine Learning Models Predicted Resource Needs with 94 Percent Accuracy
Machine learning models have shown a significant ability to predict resource requirements, reaching an accuracy of 94 percent. This capability provides organisations with tools for improved planning and allocation of resources and further highlights a growing tendency to use data to influence decisions. Yet this high accuracy needs careful evaluation. It is possible that when the input data is not evenly distributed, it may lead to inflated accuracy results that do not hold true in all situations. Ensuring the data is of high quality, and that the models are updated frequently, will be critical if the predictions are to remain valuable and consistent. While machine learning has obvious potential in optimising how companies use resources, critical evaluation and continuous development are vital for making these technological advances work well in the real world.
Machine learning models used in the study reportedly achieved 94 percent accuracy when predicting resource requirements. This high level of precision could lead to considerable cost savings, suggesting that these types of models can be directly correlated to improved financial performance, however that does not always mean that data-driven approaches equate to higher profit.
Even with high accuracy scores, the performance of these systems relies on quality input data. Flaws or biases present in the dataset may reduce the reliability of results. This highlights how crucial sound data governance practices are when making predictions.
It was also noted that these machine learning systems refine their models based on real outcomes, so future predictions improve over time. This adaptability could enable better resource management, however it needs continuous oversight and fine-tuning to remain reliable.
The study also claims that precise resource predictions led to big reductions in waste. These tools seem to align inventory with actual needs, preventing costly overstock problems, but also raising the question of understocking and if the cost savings come at a cost of production slowdowns.
One aspect that was also worth noting, was that these machine learning tools might have wider potential in various sectors. While impressive results were highlighted in the study, it is not yet clear if these models are genuinely a "universal" tool or just work well in a limited set of environments.
The use of machine learning to predict resources also highlighted data silos within an organisation, as effective predictions require data from different areas, indicating that collaboration between separate teams is vital for good results and perhaps suggesting a more general rethink of how different teams interact together within the company.
Companies that use machine learning for resource prediction also saw quicker decision-making speeds. This allows them to react faster to changes in the market and operational issues, but it could also imply that it has changed traditional hierarchy, where speed of delivery becomes more important than internal communication and broader considerations.
Interestingly, while the models provided helpful insights, some staff were initially doubtful about the change, highlighting the internal resistance many companies face when moving to data-driven solutions and highlighting that data might be used to promote more top-down solutions at a cost to a collaborative environment.
When assessing the cost of using machine learning tools against the benefits of prediction, it appears that there is a trade-off that needs to be carefully weighed. Companies have to balance initial spending on technology with long term savings, highlighting that data is not always a cost effective alternative and there may be diminishing returns, particularly at smaller scale or more complicated businesses.
Finally, the use of these AI models also poses ethical concerns over data privacy and autonomy when it comes to making decisions. As AI plays more of a predictive role, the possibility of "black box" style decision processes should be considered before implementation, ensuring that ethics is placed as an important factor, and not just cost cutting exercises.
Streamline customs compliance and documentation with AI-powered assistance. tradeclear.tech revolutionizes trade processes. (Get started for free)
More Posts from tradeclear.tech: